Our research team is pleased to announce a bi-weekly webinar series, every Friday 1 pm U.S. Eastern Time (unless otherwise specified).
Zoom ID: https://cuboulder.zoom.us/j/2251625831
Password: i-aim
Seminar information can be found below.
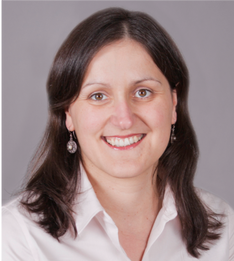
October 16th, 2:30pm EST-3:30pm EST
Maša Prodanović , University of Texas at Austin
"Connecting Data, Algorithms and Community in Digital Rock Physics"
Abstract:
Recent advances in high-resolution imaging techniques have provided a wealth of 2D and 3D datasets that reveal the microstructure of rocks and soil on scales ranging from nanometers to centimeters. Imaging in combination with simulations allow investigating non-linear flow and mechanical phenomena within the pore spaces otherwise impervious to laboratory measurements. Significant scientific opportunities can be realized by developing methods that study transport behavior within individual images of rock samples (framework popularly called digital rock physics) and considering statistical approaches to aggregate observations from many different samples and across different scales. However, in the excitement over imaging technologies that provide fantastic levels of detail, the challenges of interpreting the torrent of produced data are overlooked. The barrier to scientific progress is not acquiring data, but automation, that is enabling and maintaining a data sharing and computational environment that enables analyses, simulations, and visualizations required to link curated imaged data across spatial and temporal scales efficiently. I first address data management. The Digital Rocks Portal (https://www.digitalrocksportal.org) is the first platform of its kind for management of images of rock microstructure. It allows preservation, curation, visualization and basic analysis of images of porous materials (and experiments on them). The portal is implemented within the reliable, 24/7 maintained High Performance Computing Infrastructure supported by the Texas Advanced Computing Center at the University of Texas at Austin. I overview existing features of the portal as well as future steps in creating data-centric computing environment: i.e. cyberinfrastructure that connects data, algorithms (including traditional simulation as well as machine/deep learning) and people in a scalable way. I secondly work in my group on the application of convolutional neural network (CNN) algorithms to predicting permeability of a rock sample. Such algorithms require a lot of data in order to train. Once CNN is trained it has a promise to cut down 8+ hours simulation time down to seconds. We trained different transformations to select the most suitable mapping technique from pore scale geometry descriptors to the flow simulation using lattice Boltzmann method. The simulation was trained in granular material (sphere pack) and then trained in granular materials and sandstones. I finally discuss some outstanding problems in digital rocks community. Last but not the least, it is important to note that the data environment I describe her is directly applicable to estimating macroscopic properties based on 3D images of other complex/porous structures such as those in materials science (foams), chemical engineering (fuel cells), and medicine (bones, micro-vascular networks).
About the Speaker:
Maša Prodanović has been an associate professor at the Hildebrand Department of Petroleum and Geosystems Engineering, The University of Texas at Austin since September 2016. She holds a Bachelor of Science in Applied Mathematics from the University of Zagreb, Croatia and a PhD in Applied Mathematics and Statistics from Stony Brook University, New York, USA. Prior to her current post she has held an assistant professor position 2010-2016, a Research Associate position in the Center for Petroleum and Geosystems Engineering (UT Austin) 2007-2010, and prestigious J. T. Oden Postdoctoral Fellowship at the Institute of Computational Engineering and Sciences 2005-2007. Her research interests include multiphase flow and image‐based porous media characterization especially applied to heterogeneous porous media, pore network models, shale gas flow, particulate flow and formation damage, sediment mechanics, fracturing and ferrohydrodynamics. Most recently she received SPE Formation Evaluation regional award for development of Digital Rocks Portal in 2019, Texas 10 (top faculty) and Stony Brook 40 Under Forty awards in 2017, SPE Faculty Innovative Teaching Award in 2014 and Interpore Procter & Gamble Research Award for Porous Media Research in 2014. She organized and instructed internationally multiple short courses on image analysis in porous media.
Youtube Video: https://www.youtube.com/watch?v=SjXdKcH0dc4
Maša Prodanović , University of Texas at Austin
"Connecting Data, Algorithms and Community in Digital Rock Physics"
Abstract:
Recent advances in high-resolution imaging techniques have provided a wealth of 2D and 3D datasets that reveal the microstructure of rocks and soil on scales ranging from nanometers to centimeters. Imaging in combination with simulations allow investigating non-linear flow and mechanical phenomena within the pore spaces otherwise impervious to laboratory measurements. Significant scientific opportunities can be realized by developing methods that study transport behavior within individual images of rock samples (framework popularly called digital rock physics) and considering statistical approaches to aggregate observations from many different samples and across different scales. However, in the excitement over imaging technologies that provide fantastic levels of detail, the challenges of interpreting the torrent of produced data are overlooked. The barrier to scientific progress is not acquiring data, but automation, that is enabling and maintaining a data sharing and computational environment that enables analyses, simulations, and visualizations required to link curated imaged data across spatial and temporal scales efficiently. I first address data management. The Digital Rocks Portal (https://www.digitalrocksportal.org) is the first platform of its kind for management of images of rock microstructure. It allows preservation, curation, visualization and basic analysis of images of porous materials (and experiments on them). The portal is implemented within the reliable, 24/7 maintained High Performance Computing Infrastructure supported by the Texas Advanced Computing Center at the University of Texas at Austin. I overview existing features of the portal as well as future steps in creating data-centric computing environment: i.e. cyberinfrastructure that connects data, algorithms (including traditional simulation as well as machine/deep learning) and people in a scalable way. I secondly work in my group on the application of convolutional neural network (CNN) algorithms to predicting permeability of a rock sample. Such algorithms require a lot of data in order to train. Once CNN is trained it has a promise to cut down 8+ hours simulation time down to seconds. We trained different transformations to select the most suitable mapping technique from pore scale geometry descriptors to the flow simulation using lattice Boltzmann method. The simulation was trained in granular material (sphere pack) and then trained in granular materials and sandstones. I finally discuss some outstanding problems in digital rocks community. Last but not the least, it is important to note that the data environment I describe her is directly applicable to estimating macroscopic properties based on 3D images of other complex/porous structures such as those in materials science (foams), chemical engineering (fuel cells), and medicine (bones, micro-vascular networks).
About the Speaker:
Maša Prodanović has been an associate professor at the Hildebrand Department of Petroleum and Geosystems Engineering, The University of Texas at Austin since September 2016. She holds a Bachelor of Science in Applied Mathematics from the University of Zagreb, Croatia and a PhD in Applied Mathematics and Statistics from Stony Brook University, New York, USA. Prior to her current post she has held an assistant professor position 2010-2016, a Research Associate position in the Center for Petroleum and Geosystems Engineering (UT Austin) 2007-2010, and prestigious J. T. Oden Postdoctoral Fellowship at the Institute of Computational Engineering and Sciences 2005-2007. Her research interests include multiphase flow and image‐based porous media characterization especially applied to heterogeneous porous media, pore network models, shale gas flow, particulate flow and formation damage, sediment mechanics, fracturing and ferrohydrodynamics. Most recently she received SPE Formation Evaluation regional award for development of Digital Rocks Portal in 2019, Texas 10 (top faculty) and Stony Brook 40 Under Forty awards in 2017, SPE Faculty Innovative Teaching Award in 2014 and Interpore Procter & Gamble Research Award for Porous Media Research in 2014. She organized and instructed internationally multiple short courses on image analysis in porous media.
Youtube Video: https://www.youtube.com/watch?v=SjXdKcH0dc4
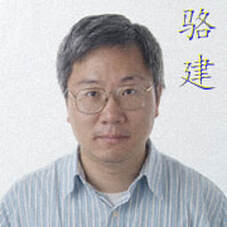
October 23rd, 1 pm EDT- 2 pm EDT:
Jian Luo, UCSD
"Deciphering the Grain Boundary Genome and Selected Other Topics Related to Data-Driven Materials Discovery"
Abstract:
A piece of ice melts at 0°C, but a nanometer-thick surface layer of the ice can melt at tens of degrees below zero. This phenomenon, known as “premelting,” was first recognized by Michael Faraday. Materials scientists have discovered that interfaces in engineered materials can exhibit more complex phase-like behaviors, which can affect the fabrication and properties of a broad range of metallic and ceramic materials. Specifically, recent studies of 2-D grain-boundary (GB) phases (also called “complexions”) shed light on several long-standing mysteries in materials science, including the atomic-level mechanisms of solid-state activated sintering [JACerS 95:2358 (2012)] and GB embrittlement [Science 333: 1730 (2011) & 358:97 (2017); Nature Comm. 9:2764 (2018)]. Since bulk phase diagrams are arguably one of the most useful tools for materials design, it is conceived that GB “phase” (complexion) diagrams can be developed as a useful materials science tool [see, e.g., Acta 20:268 (2016) & 130:329 (2017); Scripta 130:165 (2017) & 158:11 (2019); PRL 120: 085702 (2018)]. Recently genetic algorithm-guided deep learning is used to predict GB properties as a function of five crystallographic (macroscopic) degrees of freedom plus temperature and composition in a 7-D space [Materials Today 38:49 (2020)]. Analogous 2-D surface phases have also been studied and utilized to improve the performance of various materials for energy-related applications, including batteries, supercapacitors, and oxygen-ion conductors [review: Energy Storage Materials 21:50 (2019)].
To explore potential collaborative opportunities, I will also briefly discuss two other on-going studies related to “Data Science for Materials Discovery”, namely (i) flash [viewpoint: Scripta 146:260 (2018)] and other ultrafast [Acta 125:465 (2017); Scripta 142:79 (2018); Science 386:521 (2020)] sintering technologies that can densify ceramics in seconds and (ii) discoveries of new classes of high-entropy ceramics (HECs), including: high-entropy diborides as a new class of ultra-high temperature ceramics [Sci. Rep. 6:37946 (2016)], high-entropy monoborides as new class of superhard materials [Scripta 189:101 (2020)], high-entropy perovskites [Scripta 142:116 (2018)], YSZ-like high-entropy fluorite oxides [J. Eu. Ceram. Soc. 38:3578 (2018)], and a high-entropy silicide [J Materiomics 5:337 (2019)], as well as single-phase high-entropy intermetallic compounds that bridge high-entropy alloys and ceramics [Sci. Bull. 64:856 (2019)]. We recently propose to expand HECs to “compositionally-complex ceramics (CCCs),” where medium-entropy compositions can often outperform their high-entropy counterparts [Perspective: J. Mater. Sci. 55: 9812 (2020)]. On the one hand, we may utilize the ultrafast sintering technologies developed in Topic (i) for high-throughput materials fabrication to support data-driven materials discovery. On the other hand, the HECs and CCCs discovered in Topic (ii) serve as an ideal platform where data-driven materials discovery is critically needed.
About the Speaker:
Jian Luo graduated from Tsinghua University with dual Bachelor's degrees: one in Materials Science and Engineering and another in Electronics and Computer Technology. After receiving his M.S. and Ph.D. degrees from M.I.T., Luo worked in the industry for more than two years with Lucent Technologies and OFS. In 2003, he joined the Clemson faculty, where he served as an Assistant/Associate/Full Professor of Materials Science and Engineering. In 2013, he moved to UCSD as a Professor of NanoEngineering and Materials Science and Engineering. He received an NSF CAREER award in 2005 (from the Ceramics program) and an AFOSR Young Investigator award in 2007 (from the Metallic Materials program). Professor Luo is a Vannevar Bush Faculty Fellow (2014-2019) and a Fellow of the American Ceramic Society (2016-). Recently, he was selected as one of the TMS 2019 Brimacombe Medalists.
Youtube Video proprietary - please contact speaker for questions.
Jian Luo, UCSD
"Deciphering the Grain Boundary Genome and Selected Other Topics Related to Data-Driven Materials Discovery"
Abstract:
A piece of ice melts at 0°C, but a nanometer-thick surface layer of the ice can melt at tens of degrees below zero. This phenomenon, known as “premelting,” was first recognized by Michael Faraday. Materials scientists have discovered that interfaces in engineered materials can exhibit more complex phase-like behaviors, which can affect the fabrication and properties of a broad range of metallic and ceramic materials. Specifically, recent studies of 2-D grain-boundary (GB) phases (also called “complexions”) shed light on several long-standing mysteries in materials science, including the atomic-level mechanisms of solid-state activated sintering [JACerS 95:2358 (2012)] and GB embrittlement [Science 333: 1730 (2011) & 358:97 (2017); Nature Comm. 9:2764 (2018)]. Since bulk phase diagrams are arguably one of the most useful tools for materials design, it is conceived that GB “phase” (complexion) diagrams can be developed as a useful materials science tool [see, e.g., Acta 20:268 (2016) & 130:329 (2017); Scripta 130:165 (2017) & 158:11 (2019); PRL 120: 085702 (2018)]. Recently genetic algorithm-guided deep learning is used to predict GB properties as a function of five crystallographic (macroscopic) degrees of freedom plus temperature and composition in a 7-D space [Materials Today 38:49 (2020)]. Analogous 2-D surface phases have also been studied and utilized to improve the performance of various materials for energy-related applications, including batteries, supercapacitors, and oxygen-ion conductors [review: Energy Storage Materials 21:50 (2019)].
To explore potential collaborative opportunities, I will also briefly discuss two other on-going studies related to “Data Science for Materials Discovery”, namely (i) flash [viewpoint: Scripta 146:260 (2018)] and other ultrafast [Acta 125:465 (2017); Scripta 142:79 (2018); Science 386:521 (2020)] sintering technologies that can densify ceramics in seconds and (ii) discoveries of new classes of high-entropy ceramics (HECs), including: high-entropy diborides as a new class of ultra-high temperature ceramics [Sci. Rep. 6:37946 (2016)], high-entropy monoborides as new class of superhard materials [Scripta 189:101 (2020)], high-entropy perovskites [Scripta 142:116 (2018)], YSZ-like high-entropy fluorite oxides [J. Eu. Ceram. Soc. 38:3578 (2018)], and a high-entropy silicide [J Materiomics 5:337 (2019)], as well as single-phase high-entropy intermetallic compounds that bridge high-entropy alloys and ceramics [Sci. Bull. 64:856 (2019)]. We recently propose to expand HECs to “compositionally-complex ceramics (CCCs),” where medium-entropy compositions can often outperform their high-entropy counterparts [Perspective: J. Mater. Sci. 55: 9812 (2020)]. On the one hand, we may utilize the ultrafast sintering technologies developed in Topic (i) for high-throughput materials fabrication to support data-driven materials discovery. On the other hand, the HECs and CCCs discovered in Topic (ii) serve as an ideal platform where data-driven materials discovery is critically needed.
About the Speaker:
Jian Luo graduated from Tsinghua University with dual Bachelor's degrees: one in Materials Science and Engineering and another in Electronics and Computer Technology. After receiving his M.S. and Ph.D. degrees from M.I.T., Luo worked in the industry for more than two years with Lucent Technologies and OFS. In 2003, he joined the Clemson faculty, where he served as an Assistant/Associate/Full Professor of Materials Science and Engineering. In 2013, he moved to UCSD as a Professor of NanoEngineering and Materials Science and Engineering. He received an NSF CAREER award in 2005 (from the Ceramics program) and an AFOSR Young Investigator award in 2007 (from the Metallic Materials program). Professor Luo is a Vannevar Bush Faculty Fellow (2014-2019) and a Fellow of the American Ceramic Society (2016-). Recently, he was selected as one of the TMS 2019 Brimacombe Medalists.
Youtube Video proprietary - please contact speaker for questions.
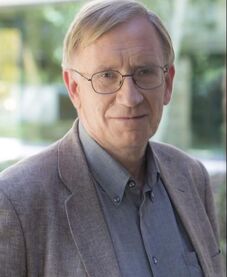
November 6 (Friday); 1 pm EST- 2 pm EST (10am -- 11am Pacific Time):
Gunnar Carlson, Stanford University
"Topological Data Analysis and Applications"
Abstract:
Topological data analysis is a rapidly developing field within mathematics and statistics. It constructs mathematical notions of shape, and ways of measuring shape. I will discuss the methods, with examples to a number of areas of the sciences and engineering.
About the Speaker:
Gunnar Carlsson received his doctoral degree from Stanford, and has taught at University of Chicago, University of California at San Diego, Princeton University, and since 1991 at Stanford University. He has worked within the area of topology, the mathematical study of shape. Since 2000, he has been working on adapting topological ideas to the study of large and complex data sets. He led a multi-university DARPA initiative on this subject from 2005 to 2010. He co-founded the company Ayasdi in 2008, which has been commercializing the research.
Youtube Video: https://www.youtube.com/watch?v=7UqRaTYTcsg
Gunnar Carlson, Stanford University
"Topological Data Analysis and Applications"
Abstract:
Topological data analysis is a rapidly developing field within mathematics and statistics. It constructs mathematical notions of shape, and ways of measuring shape. I will discuss the methods, with examples to a number of areas of the sciences and engineering.
About the Speaker:
Gunnar Carlsson received his doctoral degree from Stanford, and has taught at University of Chicago, University of California at San Diego, Princeton University, and since 1991 at Stanford University. He has worked within the area of topology, the mathematical study of shape. Since 2000, he has been working on adapting topological ideas to the study of large and complex data sets. He led a multi-university DARPA initiative on this subject from 2005 to 2010. He co-founded the company Ayasdi in 2008, which has been commercializing the research.
Youtube Video: https://www.youtube.com/watch?v=7UqRaTYTcsg
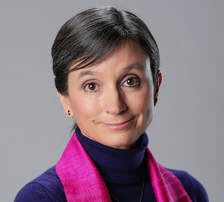
November 20
Kathryn Hess, EPFL
"From Trees to Barcodes and Back Again"
Abstract:
Methods of topological data analysis have been successfully applied in a wide range of fields to provide useful summaries of the structure of complex data sets in terms of topological descriptors, such as persistence diagrams. While there are many powerful techniques for computing topological descriptors, the inverse problem, i.e., recovering the input data from topological descriptors, has proved to be challenging.
In this talk I will focus on the Topological Morphology Descriptor (TMD), which assigns a persistence diagram to any tree embedded in Euclidean space, and a sort of stochastic inverse to the TMD, the Topological Neuron Synthesis (TNS) algorithm. I will provide an overview of the TMD and the TNS and then describe the results of our theoretical and computational analysis of their behavior and properties, in which symmetric groups play a key role. In particular, I will specify the extent to which the TNS provides an inverse to the TMD.
This is joint work with Adélie Garin and Lida Kanari, based on earlier collaborations led by Lida.
About the Speaker:
Kathryn Hess is a professor of mathematics and life sciences at the EPFL. She received her PhD from MIT and held positions at the universities of Stockholm, Nice, and Toronto before moving to the EPFL. Her research focuses on algebraic topology and its applications, primarily in the life sciences, but also in materials science. On the applied side, she has elaborated methods based on topological data analysis for high-throughput screening of nanoporous crystalline materials, classification and synthesis of neuron morphologies, and classification of neuronal network dynamics. She has also developed and applied innovative topological approaches to network theory, leading to a powerful, parameter-free mathematical framework relating the activity of a neural network to its underlying structure, both locally and globally.
In 2016 she was elected to Swiss Academy of Engineering Sciences and was named a fellow of the American Mathematical Society and a distinguished speaker of the European Mathematical Society in 2017.
Youtube Video: https://youtu.be/n0NbDaV0gU4
Kathryn Hess, EPFL
"From Trees to Barcodes and Back Again"
Abstract:
Methods of topological data analysis have been successfully applied in a wide range of fields to provide useful summaries of the structure of complex data sets in terms of topological descriptors, such as persistence diagrams. While there are many powerful techniques for computing topological descriptors, the inverse problem, i.e., recovering the input data from topological descriptors, has proved to be challenging.
In this talk I will focus on the Topological Morphology Descriptor (TMD), which assigns a persistence diagram to any tree embedded in Euclidean space, and a sort of stochastic inverse to the TMD, the Topological Neuron Synthesis (TNS) algorithm. I will provide an overview of the TMD and the TNS and then describe the results of our theoretical and computational analysis of their behavior and properties, in which symmetric groups play a key role. In particular, I will specify the extent to which the TNS provides an inverse to the TMD.
This is joint work with Adélie Garin and Lida Kanari, based on earlier collaborations led by Lida.
About the Speaker:
Kathryn Hess is a professor of mathematics and life sciences at the EPFL. She received her PhD from MIT and held positions at the universities of Stockholm, Nice, and Toronto before moving to the EPFL. Her research focuses on algebraic topology and its applications, primarily in the life sciences, but also in materials science. On the applied side, she has elaborated methods based on topological data analysis for high-throughput screening of nanoporous crystalline materials, classification and synthesis of neuron morphologies, and classification of neuronal network dynamics. She has also developed and applied innovative topological approaches to network theory, leading to a powerful, parameter-free mathematical framework relating the activity of a neural network to its underlying structure, both locally and globally.
In 2016 she was elected to Swiss Academy of Engineering Sciences and was named a fellow of the American Mathematical Society and a distinguished speaker of the European Mathematical Society in 2017.
Youtube Video: https://youtu.be/n0NbDaV0gU4
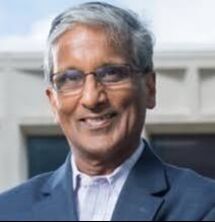
December 18
Krishna Rajan, SUNY Buffalo
"Data Driven Discovery of Pathways for Materials Design"
Abstract:
The materials science community has in the last decade has used the biological metaphor of “genomics” to guide materials discovery in many ways. However, to fully exploit the challenge of translating what we learn from fundamental materials discovery to exploring multiscale phenomena, require us to be willing to look for connections in the complex network of relationships that govern materials behavior. In this lecture we include examples of harnessing informatics techniques for mapping diverse information and discovering connections between structure and functionality in materials.
About the Speaker:
Krishna Rajan is the SUNY Distinguished Professor and Erich Bloch Chair in the Department of Materials Design and Innovation at the University at Buffalo. Professor Rajan is the leading proponent of the field of Materials Informatics. His research is centered on the application of information science and data intensive methodologies for the discovery, characterization and modeling of materials. Along with computational studies, he is a leader in the field of advancing quantitative methods for the interpretation of nanoscale chemical imaging techniques such as, atom probe tomography.
Youtube Video: https://www.youtube.com/watch?v=2QZJlXtaWNg
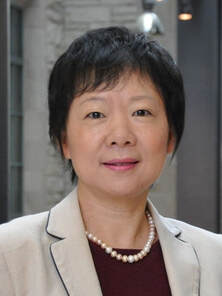
February 19, 2021
Wei Chen, Northwestern University
"Data-Driven Design of Microstructural Material Systems"
Abstract:
Design of advanced material systems imposes challenges in integrating knowledge and representation from multiple disciplines and domains such as materials, manufacturing, structural mechanics, and design optimization.Data-driven machine learning and computational design methods provide a seamless integration of predictive materials modeling, manufacturing, and design optimization to enable the accelerated design and deployment of advanced materials systems. In this talk, we will introduce the state-of-the-art data science methods for designing heterogeneous nano- and microstructural materials and metamaterial systems such as polymer nanocomposites, light-weight composite structures, functional microelectronics, and solar cells. Research developments in microstructure characterization and reconstruction, machine learning of key microstructure features, and data-driven Bayesian optimization using mixed-variable Gaussian process models, will be introduced. Challenges and opportunities in designing engineered material systems will be discussed.
About the Speaker:
Wei Chen is the Wilson-Cook Professor in Engineering Design and Chair of Department of Mechanical Engineering at Northwestern University. Directing the Integrated DEsign Automation Laboratory (IDEAL- http://ideal.mech.northwestern.edu/), her current research involves issues such as simulation-based design under uncertainty; model validation and uncertainty quantification; data science in design and advanced manufacturing; stochastic multiscale analysis and materials design; design of metamaterials; multidisciplinary design optimization; consumer choice modeling and decision-based design. She is a member of the National Academy of Engineering (NAE) and currently serving as the Editor-in-chief of the ASME Journal of Mechanical Design and the President of the International Society of Structural and Multidisciplinary Design (ISSMO). Dr. Chen was the recipient of the 2015 ASME Design Automation Award and 1996 NSF Faculty Career Award. She received her Ph.D. from the Georgia Institute of Technology in 1995.
Youtube Video: https://www.youtube.com/watch?v=podC8P3daoU
Wei Chen, Northwestern University
"Data-Driven Design of Microstructural Material Systems"
Abstract:
Design of advanced material systems imposes challenges in integrating knowledge and representation from multiple disciplines and domains such as materials, manufacturing, structural mechanics, and design optimization.Data-driven machine learning and computational design methods provide a seamless integration of predictive materials modeling, manufacturing, and design optimization to enable the accelerated design and deployment of advanced materials systems. In this talk, we will introduce the state-of-the-art data science methods for designing heterogeneous nano- and microstructural materials and metamaterial systems such as polymer nanocomposites, light-weight composite structures, functional microelectronics, and solar cells. Research developments in microstructure characterization and reconstruction, machine learning of key microstructure features, and data-driven Bayesian optimization using mixed-variable Gaussian process models, will be introduced. Challenges and opportunities in designing engineered material systems will be discussed.
About the Speaker:
Wei Chen is the Wilson-Cook Professor in Engineering Design and Chair of Department of Mechanical Engineering at Northwestern University. Directing the Integrated DEsign Automation Laboratory (IDEAL- http://ideal.mech.northwestern.edu/), her current research involves issues such as simulation-based design under uncertainty; model validation and uncertainty quantification; data science in design and advanced manufacturing; stochastic multiscale analysis and materials design; design of metamaterials; multidisciplinary design optimization; consumer choice modeling and decision-based design. She is a member of the National Academy of Engineering (NAE) and currently serving as the Editor-in-chief of the ASME Journal of Mechanical Design and the President of the International Society of Structural and Multidisciplinary Design (ISSMO). Dr. Chen was the recipient of the 2015 ASME Design Automation Award and 1996 NSF Faculty Career Award. She received her Ph.D. from the Georgia Institute of Technology in 1995.
Youtube Video: https://www.youtube.com/watch?v=podC8P3daoU
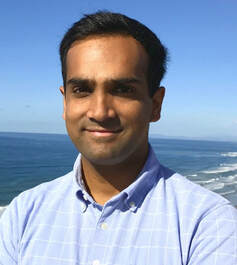
March 19, 2021
Arun Kumar, UCSD
"Cerebro: A Layered Data Platform for Scalable Deep Learning"
Abstract:
Deep learning (DL) is gaining popularity across many domains thanks to tools such as PyTorch and easier access to GPUs. But building large-scale DL applications is still too resource-intensive and painful for all but the big tech firms. A key reason for this pain is the expensive model selection process needed to get DL to work well. This process requires exploring alternative representations of signals from various modalities (e.g., materials imaging and simulation time series), neural architecture tuning (e.g., physics-inspired architectures), and hyperparameter tuning (knobs to control underfitting vs overfitting). Existing DL systems treat this process as an afterthought, leading to massive resource wastage and a usability mess. To tackle these issues, we present our vision of a first-of-its-kind data platform for scalable DL, Cerebro, inspired by lessons from the database world (https://adalabucsd.github.io/cerebro.html). We elevate the DL model selection process with higher-level APIs already inherent in practice and devise a series of novel multi-query optimization techniques to substantially raise resource efficiency. In turn, this can help improve scalability, reduce resource costs and energy usage, and improve DL user productivity. This talk will present our system design rationales and architecture, our recent research and empirical results, a discussion on how Cerebro is being applied to use cases in public health, its potential for enhancing the use of large-scale DL in materials science and engineering, and our future research plans.
Overview paper (9 pg): http://cidrdb.org/cidr2021/papers/cidr2021_paper25.pdf; Short video (10 min): https://www.youtube.com/watch?v=8QfMvdlmdic
About the Speaker:
Arun Kumar is an Assistant Professor in the Department of Computer Science and Engineering and the Halicioglu Data Science Institute at the University of California, San Diego (https://adalabucsd.github.io). He is a member of the Database Lab and Center for Networked Systems and an affiliate member of the AI Group. His primary research interests are in data management and systems for machine learning/artificial intelligence-based data analytics. Systems and ideas based on his research have been released as part of the Apache MADlib open-source library, shipped as part of products from Cloudera, IBM, Oracle, and Pivotal, and used internally by Facebook, Google, LogicBlox, Microsoft, and other companies. He is a recipient of two SIGMOD research paper awards, a SIGMOD Research Highlight Award, three distinguished reviewer awards from SIGMOD/VLDB, the PhD dissertation award from UW-Madison CS, an NSF CAREER Award, a Hellman Fellowship, a UCSD oSTEM Faculty of the Year Award, and research award gifts from Google, Oracle, and VMware.
Youtube Video: https://www.youtube.com/watch?v=ERKyvLT4Wik
Arun Kumar, UCSD
"Cerebro: A Layered Data Platform for Scalable Deep Learning"
Abstract:
Deep learning (DL) is gaining popularity across many domains thanks to tools such as PyTorch and easier access to GPUs. But building large-scale DL applications is still too resource-intensive and painful for all but the big tech firms. A key reason for this pain is the expensive model selection process needed to get DL to work well. This process requires exploring alternative representations of signals from various modalities (e.g., materials imaging and simulation time series), neural architecture tuning (e.g., physics-inspired architectures), and hyperparameter tuning (knobs to control underfitting vs overfitting). Existing DL systems treat this process as an afterthought, leading to massive resource wastage and a usability mess. To tackle these issues, we present our vision of a first-of-its-kind data platform for scalable DL, Cerebro, inspired by lessons from the database world (https://adalabucsd.github.io/cerebro.html). We elevate the DL model selection process with higher-level APIs already inherent in practice and devise a series of novel multi-query optimization techniques to substantially raise resource efficiency. In turn, this can help improve scalability, reduce resource costs and energy usage, and improve DL user productivity. This talk will present our system design rationales and architecture, our recent research and empirical results, a discussion on how Cerebro is being applied to use cases in public health, its potential for enhancing the use of large-scale DL in materials science and engineering, and our future research plans.
Overview paper (9 pg): http://cidrdb.org/cidr2021/papers/cidr2021_paper25.pdf; Short video (10 min): https://www.youtube.com/watch?v=8QfMvdlmdic
About the Speaker:
Arun Kumar is an Assistant Professor in the Department of Computer Science and Engineering and the Halicioglu Data Science Institute at the University of California, San Diego (https://adalabucsd.github.io). He is a member of the Database Lab and Center for Networked Systems and an affiliate member of the AI Group. His primary research interests are in data management and systems for machine learning/artificial intelligence-based data analytics. Systems and ideas based on his research have been released as part of the Apache MADlib open-source library, shipped as part of products from Cloudera, IBM, Oracle, and Pivotal, and used internally by Facebook, Google, LogicBlox, Microsoft, and other companies. He is a recipient of two SIGMOD research paper awards, a SIGMOD Research Highlight Award, three distinguished reviewer awards from SIGMOD/VLDB, the PhD dissertation award from UW-Madison CS, an NSF CAREER Award, a Hellman Fellowship, a UCSD oSTEM Faculty of the Year Award, and research award gifts from Google, Oracle, and VMware.
Youtube Video: https://www.youtube.com/watch?v=ERKyvLT4Wik
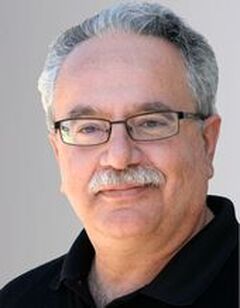
April 2, 2021
Yannis Kevrekidis, Johns Hopkins University
"Manifold Learning for Free Energy Surface Exploration"
Abstract:
Here follows an informal introduction - You have an MD or Monte Carlo code; you believe that there exists a coarse grained, effective free energy surface; and you want to either (a) approximately construct it or (b) perform a task on it, like finding a transition state between two minima. How to do it with the least amount of fine scale/atomistic computation ? I will discuss the equation free/variable free approach to this, that we have been working on since 2000 (see, e.g. [1-3] sampling over the years). It constitutes an integration of "the best atomistic simulation you can do" with manifold learning, numerical analysis and system identification - you all know/recognize the pieces, and the area has been "booming" recently. In particular, I will focus on our recent "intrinsic map dynamics", (iMapD) [4] and its current extensions. Looking for people to play with!
Key references:
[1] "Coarse molecular dynamics of a peptide fragment: free energy, kinetics and long time dynamics computations"
G. Hummer and I.G.K., J. Chem. Phys. 118(23) pp. 10762-10773 (2003)
[2] "Coarse-grained kinetic computations of rare events: application to micelle formation"
D. I. Kopelevich, A. Z. Panagiotopoulos and I. G. Kevrekidis, J. Chem. Phys. 122 044908 (2005)
[3] "Exploration of effective potential landscapes using coarse reverse integration"
T. A. Frewen, G. Hummer and I. G. Kevrekidis, J. Chem. Phys. 131 134104 (2009)
lots of other "stuff" and then
[4] "iMapD: intrinsic Map Dynamics exploration for uncharted effective free energy landscapes"
E. Chiavazzo, Ronald R. Coifman, Roberto Covino, C. William Gear, Anastasia S. Georgiou, Gerhard Hummer, Ioannis G. Kevrekidis, PNAS 114(28) pp. E5494-5503 (2017)
About the Speaker:
Yannis Kevrekidis is the Bloomberg Distinguished Professor in the Departments of Chemical and Biomolecular Engineering, Department of Applied Mathematics and Statistics, and in the School of Medicine at Johns Hopkins University. He is a member of the National Academy of Arts and Sciences and has been a Packard Fellow, an NSF Presidential Young Investigator, and a Guggenheim Fellow. He holds the Colburn, the Wilhelm, and the Computing in Chemical Engineering awards of the AIChE; the Crawford Prize and the W.T. and Idalia Reid Prize of SIAM; and a Senior Humboldt prize. He has been the Gutzwiller Fellow at the Max Planck Institute for the Physics of Complex Systems in Dresden and a Rothschild Distinguished Visitor at the Newton Institute at Cambridge University, and is currently a senior Hans Fischer Fellow at IAS-TUM in Munich and an Einstein Visiting Fellow at FU/Zuse Institut Berlin. In 2015, he was elected a corresponding member of the Academy of Athens. He also holds a career Teaching Award from the School of Engineering at Princeton University.
Youtube Video: https://www.youtube.com/watch?v=ybV4z5M30PM
Yannis Kevrekidis, Johns Hopkins University
"Manifold Learning for Free Energy Surface Exploration"
Abstract:
Here follows an informal introduction - You have an MD or Monte Carlo code; you believe that there exists a coarse grained, effective free energy surface; and you want to either (a) approximately construct it or (b) perform a task on it, like finding a transition state between two minima. How to do it with the least amount of fine scale/atomistic computation ? I will discuss the equation free/variable free approach to this, that we have been working on since 2000 (see, e.g. [1-3] sampling over the years). It constitutes an integration of "the best atomistic simulation you can do" with manifold learning, numerical analysis and system identification - you all know/recognize the pieces, and the area has been "booming" recently. In particular, I will focus on our recent "intrinsic map dynamics", (iMapD) [4] and its current extensions. Looking for people to play with!
Key references:
[1] "Coarse molecular dynamics of a peptide fragment: free energy, kinetics and long time dynamics computations"
G. Hummer and I.G.K., J. Chem. Phys. 118(23) pp. 10762-10773 (2003)
[2] "Coarse-grained kinetic computations of rare events: application to micelle formation"
D. I. Kopelevich, A. Z. Panagiotopoulos and I. G. Kevrekidis, J. Chem. Phys. 122 044908 (2005)
[3] "Exploration of effective potential landscapes using coarse reverse integration"
T. A. Frewen, G. Hummer and I. G. Kevrekidis, J. Chem. Phys. 131 134104 (2009)
lots of other "stuff" and then
[4] "iMapD: intrinsic Map Dynamics exploration for uncharted effective free energy landscapes"
E. Chiavazzo, Ronald R. Coifman, Roberto Covino, C. William Gear, Anastasia S. Georgiou, Gerhard Hummer, Ioannis G. Kevrekidis, PNAS 114(28) pp. E5494-5503 (2017)
About the Speaker:
Yannis Kevrekidis is the Bloomberg Distinguished Professor in the Departments of Chemical and Biomolecular Engineering, Department of Applied Mathematics and Statistics, and in the School of Medicine at Johns Hopkins University. He is a member of the National Academy of Arts and Sciences and has been a Packard Fellow, an NSF Presidential Young Investigator, and a Guggenheim Fellow. He holds the Colburn, the Wilhelm, and the Computing in Chemical Engineering awards of the AIChE; the Crawford Prize and the W.T. and Idalia Reid Prize of SIAM; and a Senior Humboldt prize. He has been the Gutzwiller Fellow at the Max Planck Institute for the Physics of Complex Systems in Dresden and a Rothschild Distinguished Visitor at the Newton Institute at Cambridge University, and is currently a senior Hans Fischer Fellow at IAS-TUM in Munich and an Einstein Visiting Fellow at FU/Zuse Institut Berlin. In 2015, he was elected a corresponding member of the Academy of Athens. He also holds a career Teaching Award from the School of Engineering at Princeton University.
Youtube Video: https://www.youtube.com/watch?v=ybV4z5M30PM
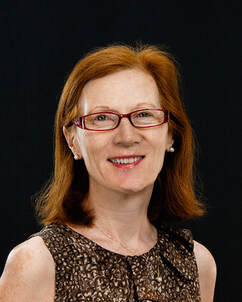
April 16, 2021
Margaret Murnane, CU Boulder
"Extracting the Structural, Mechanical, and Transport Properties of Nanostructured Materials"
Abstract:
Nanostructured materials can exhibit exotic properties that enrich the landscape of bulk materials, due to the influence of dopants, surfaces, geometry and interfaces. Moreover, at the nanometer length scale, conventional macroscopic models of materials fail to accurately describe their physical behavior. In this talk, we will discuss how ultrafast, coherent extreme ultraviolet (EUV) sources uncovered surprising new transport behavior in nanostructured systems, from nanostructures on single crystal silicon to complex metalattices. Counterintuitively we found that the thermal dissipation efficiency increases as nanoscale heat sources are packed more closely together. Combined with advanced theory, this new understanding can address current roadblocks for nano- and quantum technologies, including the development of advanced nanoelectronics and efficient thermoelectric devices.
We will also discuss how the full mechanical properties of the thinnest films being developed for advanced nanoelectronics can be measured. In some materials, as the layers are thinned, the mechanical properties can become nearly 10 times weaker than expected.
About the Speaker:
Margaret is a Fellow of JILA and a Distinguished Professor at the University of Colorado. She runs a joint, multi-disciplinary, research group with her husband, Prof. Henry Kapteyn. She received her B.S and M.S. degrees from University College Cork, Ireland, and her Ph.D. degree from UC Berkeley. Margaret, with her group and collaborators, uses coherent beams of laser and x-ray light to capture and manipulate the structure and interactions in materials at the nanoscale. She is a Fellow of the American Physical Society, and the AAAS, and a member of the National Academy of Sciences and the American Philosophical Society. In 2021, she and Henry will share the Benjamin Franklin Medal in Physics.
Youtube Video: https://www.youtube.com/watch?v=gewsNE3d6Ho
Margaret Murnane, CU Boulder
"Extracting the Structural, Mechanical, and Transport Properties of Nanostructured Materials"
Abstract:
Nanostructured materials can exhibit exotic properties that enrich the landscape of bulk materials, due to the influence of dopants, surfaces, geometry and interfaces. Moreover, at the nanometer length scale, conventional macroscopic models of materials fail to accurately describe their physical behavior. In this talk, we will discuss how ultrafast, coherent extreme ultraviolet (EUV) sources uncovered surprising new transport behavior in nanostructured systems, from nanostructures on single crystal silicon to complex metalattices. Counterintuitively we found that the thermal dissipation efficiency increases as nanoscale heat sources are packed more closely together. Combined with advanced theory, this new understanding can address current roadblocks for nano- and quantum technologies, including the development of advanced nanoelectronics and efficient thermoelectric devices.
We will also discuss how the full mechanical properties of the thinnest films being developed for advanced nanoelectronics can be measured. In some materials, as the layers are thinned, the mechanical properties can become nearly 10 times weaker than expected.
About the Speaker:
Margaret is a Fellow of JILA and a Distinguished Professor at the University of Colorado. She runs a joint, multi-disciplinary, research group with her husband, Prof. Henry Kapteyn. She received her B.S and M.S. degrees from University College Cork, Ireland, and her Ph.D. degree from UC Berkeley. Margaret, with her group and collaborators, uses coherent beams of laser and x-ray light to capture and manipulate the structure and interactions in materials at the nanoscale. She is a Fellow of the American Physical Society, and the AAAS, and a member of the National Academy of Sciences and the American Philosophical Society. In 2021, she and Henry will share the Benjamin Franklin Medal in Physics.
Youtube Video: https://www.youtube.com/watch?v=gewsNE3d6Ho
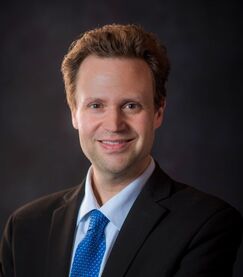
April 30, 2021
Markus Buehler, MIT
"Bioinspired AI for Hierarchical Material Design: Modeling, Design, and Manufacturing"
Abstract:
Nature produces a variety of materials with many functions, often out of simple and abundant materials, and at low energy. Such systems - examples of which include silk, bone, nacre or diatoms - provide broad inspiration for engineering. Here we explore the translation of biological composites to engineering applications, using a variety of tools including molecular modeling, AI and machine learning, and experimental synthesis and characterization. We review a series of studies focused on the mechanical behavior of materials, especially fracture, and how these phenomena can be modeled using a combination of molecular dynamics and machine learning. We also present various case studies of hierarchical material optimization using genetic algorithms, applied to 3D printed composites, protein design, and a translation of protein folding to music and back, to offer a broad bio-inspired AI-driven material synthesis platform. As an example we present a recent study in which we translated JS Bach’s Goldberg Variations into protein form, and elucidated salient features of the resulting molecular conformations and material functions.
About the Speaker:
Markus J. Buehler is the McAfee Professor of Engineering at MIT, a member of the Center for Materials Science and Engineering, and the Center for Computational Science and Engineering at the Schwarzman College of Computing. In his research, Professor Buehler pursues new modeling, design and manufacturing approaches for advanced biomaterials that offer greater resilience and a wide range of controllable properties from the nano- to the macroscale. His interests include a variety of functional material properties including mechanical, optical and biological, linking chemical features, hierarchical and multiscale structures, to assessments of material performance in the context of physiological, pathological and other extreme conditions. His methods include molecular and multiscale modeling, AI and ML, as well as experimental synthesis and characterization. His particular interest lies in the mechanics of complex hierarchical materials with features across scales, e.g. nanotubes, graphene and natural biomaterial nanostructures, especially protein materials. (Longer version: https://web.mit.edu/mbuehler/www/group/Markus_Buehler_Biography.pdf)
Youtube Video: Please contact Dr. Buehler for details (contains unpublished work).
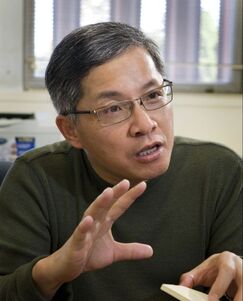
May 14, 2021
Jianwei (John) Miao, UCLA
"Beyond Crystallography: Coherent Diffractive Imaging and Atomic Electron Tomography"
Abstract:
Over the last century, crystallography has been fundamental to the development of many fields of science. However, many samples in materials science, physics, chemistry, nanoscience, geology, and biology are non-crystalline, and thus their 3D structures are not accessible by crystallography. In this talk, I will present two methods that can go beyond crystallography: coherent diffractive imaging (CDI) and atomic electron tomography (AET). I will illustrate the basic principle of CDI and present AET for 3D structure determination of crystal defects and amorphous materials at the single-atom level. I will also briefly discuss the Materials Data Bank, which archives the 3D coordinates and chemical species of individual atoms in materials without assuming crystallinity.
About the Speaker:
Miao is a professor of Physics and Astronomy and California NanoSystems Institute at UCLA. He conducted a seminal experiment of extending X-ray crystallography to non-crystalline specimens in 1999, which is known as CDI, lensless or computational microscopy. CDI methods such as ptychography and Bragg CDI have been broadly implemented using synchrotron radiation, X-ray free electron lasers, high harmonic generation, electron and light microscopy. In addition, Miao pioneered AET in 2012. He has since performed several ground-breaking AET experiments and developed 4D AET to observe crystal nucleation at atomic resolution. More recently, he led a team that determined the 3D atomic structure of an amorphous material for the first time.
Youtube Video: https://www.youtube.com/watch?v=-skmpemzejc
Jianwei (John) Miao, UCLA
"Beyond Crystallography: Coherent Diffractive Imaging and Atomic Electron Tomography"
Abstract:
Over the last century, crystallography has been fundamental to the development of many fields of science. However, many samples in materials science, physics, chemistry, nanoscience, geology, and biology are non-crystalline, and thus their 3D structures are not accessible by crystallography. In this talk, I will present two methods that can go beyond crystallography: coherent diffractive imaging (CDI) and atomic electron tomography (AET). I will illustrate the basic principle of CDI and present AET for 3D structure determination of crystal defects and amorphous materials at the single-atom level. I will also briefly discuss the Materials Data Bank, which archives the 3D coordinates and chemical species of individual atoms in materials without assuming crystallinity.
About the Speaker:
Miao is a professor of Physics and Astronomy and California NanoSystems Institute at UCLA. He conducted a seminal experiment of extending X-ray crystallography to non-crystalline specimens in 1999, which is known as CDI, lensless or computational microscopy. CDI methods such as ptychography and Bragg CDI have been broadly implemented using synchrotron radiation, X-ray free electron lasers, high harmonic generation, electron and light microscopy. In addition, Miao pioneered AET in 2012. He has since performed several ground-breaking AET experiments and developed 4D AET to observe crystal nucleation at atomic resolution. More recently, he led a team that determined the 3D atomic structure of an amorphous material for the first time.
Youtube Video: https://www.youtube.com/watch?v=-skmpemzejc
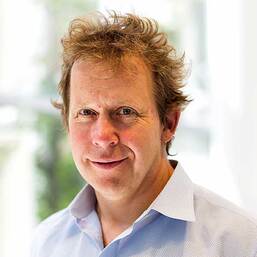
May 28, 2021
Nicholas Kotov, University of Michigan
"Graph Theoretical Descriptors for Biomimetic Nanoparticles and Fibrous Nanocomposites"
Abstract:
Descriptors based on graph theory (GT) are needed to achieve accurate representations of two classes of nanostructures for the successful application of machine learning (ML). First, a method to depict protein structure at molecular, nanoscale, and sub-microscale levels is described to predict complex formation and organization of protein-nanoparticle interfaces using several ML-algorithms. Second, a methodology to utilize GT descriptors in nanofibrous composites is developed. The computational package StructuralGT is introduced to automatically produce a GT description and structural descriptors of percolating nanoscale networks from micrographs.
About the Speaker:
Nicholas Kotov is the Irving Langmuir Distinguished Professor of Chemical Sciences and Engineering at the University of Michigan. He demonstrated that the ability to self-organize into complex structures is the unifying property of all inorganic nanostructures. He developed a family of bioinspired composite materials with a wide spectrum of properties that were previously unattainable in classical materials, such as nacre-like ultrastrong, transparent composites, enamel-like, stiff yet vibration-isolating composites, and cartilage-like membranes with high strength and ion conductance.
Youtube Video: https://youtu.be/hpz1pv0u9Ws
Nicholas Kotov, University of Michigan
"Graph Theoretical Descriptors for Biomimetic Nanoparticles and Fibrous Nanocomposites"
Abstract:
Descriptors based on graph theory (GT) are needed to achieve accurate representations of two classes of nanostructures for the successful application of machine learning (ML). First, a method to depict protein structure at molecular, nanoscale, and sub-microscale levels is described to predict complex formation and organization of protein-nanoparticle interfaces using several ML-algorithms. Second, a methodology to utilize GT descriptors in nanofibrous composites is developed. The computational package StructuralGT is introduced to automatically produce a GT description and structural descriptors of percolating nanoscale networks from micrographs.
About the Speaker:
Nicholas Kotov is the Irving Langmuir Distinguished Professor of Chemical Sciences and Engineering at the University of Michigan. He demonstrated that the ability to self-organize into complex structures is the unifying property of all inorganic nanostructures. He developed a family of bioinspired composite materials with a wide spectrum of properties that were previously unattainable in classical materials, such as nacre-like ultrastrong, transparent composites, enamel-like, stiff yet vibration-isolating composites, and cartilage-like membranes with high strength and ion conductance.
Youtube Video: https://youtu.be/hpz1pv0u9Ws
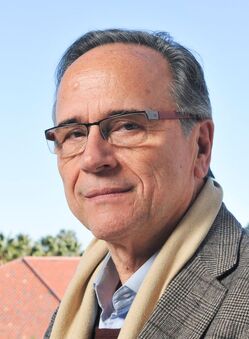
June 11, 2021
Leonidas Guibas, Stanford University
"The Particle and Wave Theories of Shape"
Abstract:
In this talk we present two very different paradigms for modeling and processing 3D shapes and their geometry. First we cover deep learning techniques for point cloud representations and introduce our PointNet and PointNet++ architectures with some applications in object detection. We also discuss how one can enforce appropriate equivariances and invariances in such networks. Then we switch to a dual setting and approach shapes via function spaces living on the shape geometry and study shape correspondences via the machinery of functional maps, showing how networks of such maps can lead to unsupervised discovery of shape structure. While these methods have largely been used in the study of macro geometry, such as that of everyday objects, applications to material geometric structures can also be interesting to pursue.
About the Speaker:
Leonidas Guibas is the Paul Pigott Professor of Computer Science (and by courtesy, Electrical Engineering) at Stanford University, where he heads the Geometric Computation group. Dr. Guibas obtained his Ph.D. from Stanford University under the supervision of Donald Knuth. His main subsequent employers were Xerox PARC, DEC/SRC, MIT, and Stanford. He is a member and past acting director of the Stanford Artificial Intelligence Laboratory and a member of the Computer Graphics Laboratory, the Institute for Computational and Mathematical Engineering (iCME) and the Bio-X program. Dr. Guibas has been elected to the US National Academy of Engineering and the American Academy of Arts and Sciences, and is an ACM Fellow, an IEEE Fellow and winner of the ACM Allen Newell award and the ICCV Helmholtz prize.
Youtube Video: Please contact Dr. Guibas for details (contains unpublished work).
Leonidas Guibas, Stanford University
"The Particle and Wave Theories of Shape"
Abstract:
In this talk we present two very different paradigms for modeling and processing 3D shapes and their geometry. First we cover deep learning techniques for point cloud representations and introduce our PointNet and PointNet++ architectures with some applications in object detection. We also discuss how one can enforce appropriate equivariances and invariances in such networks. Then we switch to a dual setting and approach shapes via function spaces living on the shape geometry and study shape correspondences via the machinery of functional maps, showing how networks of such maps can lead to unsupervised discovery of shape structure. While these methods have largely been used in the study of macro geometry, such as that of everyday objects, applications to material geometric structures can also be interesting to pursue.
About the Speaker:
Leonidas Guibas is the Paul Pigott Professor of Computer Science (and by courtesy, Electrical Engineering) at Stanford University, where he heads the Geometric Computation group. Dr. Guibas obtained his Ph.D. from Stanford University under the supervision of Donald Knuth. His main subsequent employers were Xerox PARC, DEC/SRC, MIT, and Stanford. He is a member and past acting director of the Stanford Artificial Intelligence Laboratory and a member of the Computer Graphics Laboratory, the Institute for Computational and Mathematical Engineering (iCME) and the Bio-X program. Dr. Guibas has been elected to the US National Academy of Engineering and the American Academy of Arts and Sciences, and is an ACM Fellow, an IEEE Fellow and winner of the ACM Allen Newell award and the ICCV Helmholtz prize.
Youtube Video: Please contact Dr. Guibas for details (contains unpublished work).
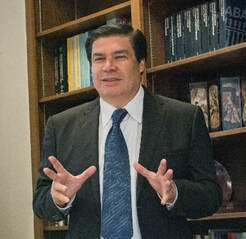
https://www.youtube.com/watch?v=t-d1dl5XRgwJune 25, 2021
Horacio Espinosa, Northwestern University
"Machine Learning for Force Field Parameterization - Application to 2D Materials"
Abstract:
The parameterization of interatomic potentials for molecular dynamics (MD) simulations has long been a highly-specialized endeavor requiring strong domain expertise and in most cases deep chemical intuition. We propose a robust approach incorporating multi-objective genetic algorithms and machine-learning-inspired protocols. Using monolayer MoSe2 as a testbed, we demonstrate the effectiveness of the proposed approach in parametrizing interatomic potentials with different levels of complexities for structural and mechanical properties in both the equilibrium and non-equilibrium regimes. In this presentation, I will show that the methodology achieves better accuracy than existing MoSe2 force fields for non-equilibrium properties including uniaxial tension and vacancy formation energies, which are crucial in probing failure of 2D materials in applications of interest, e.g., flexible electronics. Moreover, I will describe how correlation relationships, between properties of interest, can be computed and used to ascertain the impact of the functional forms, used in common interatomic potentials, and parametrization trade-offs. Finally, force field transferability will be examined in the context of heat transfer and surface stability.
About the Speaker:
Horacio D. Espinosa is the James and Nancy Farley Professor of Manufacturing and Entrepreneurship, Professor of Mechanical Engineering, and the Director of the Theoretical and Applied Mechanics Program at the McCormick School of Engineering, Northwestern University. He received his Ph.D. in Solid Mechanics from Brown University in 1992. Espinosa has made contributions in the areas of deformation and failure of materials, design of micro- and nano-systems, in situ microscopy characterization of nanomaterials, and microfluidics for single cell manipulation and analysis. He has published over 300 technical papers in these topics. Espinosa received a number of awards including the PRAGER Medal from the Society of Engineering Science, the Society for Experimental Mechanics MURRAY and SIA NEMAT NASSER Medals, and the ASME THURSTON award. He is a member of the National Academy of Engineering (NAE), foreign member of Academia Europaea, the European Academy of Arts and Sciences, the Russian Academy of Engineering, and Fellow of AAAS, ASME, SEM, and AAM. He was the President of the Society of Engineering Science in 2012 and is a member of the IUTAM General Assembly.
Youtube Video: https://www.youtube.com/watch?v=t-d1dl5XRgw
Horacio Espinosa, Northwestern University
"Machine Learning for Force Field Parameterization - Application to 2D Materials"
Abstract:
The parameterization of interatomic potentials for molecular dynamics (MD) simulations has long been a highly-specialized endeavor requiring strong domain expertise and in most cases deep chemical intuition. We propose a robust approach incorporating multi-objective genetic algorithms and machine-learning-inspired protocols. Using monolayer MoSe2 as a testbed, we demonstrate the effectiveness of the proposed approach in parametrizing interatomic potentials with different levels of complexities for structural and mechanical properties in both the equilibrium and non-equilibrium regimes. In this presentation, I will show that the methodology achieves better accuracy than existing MoSe2 force fields for non-equilibrium properties including uniaxial tension and vacancy formation energies, which are crucial in probing failure of 2D materials in applications of interest, e.g., flexible electronics. Moreover, I will describe how correlation relationships, between properties of interest, can be computed and used to ascertain the impact of the functional forms, used in common interatomic potentials, and parametrization trade-offs. Finally, force field transferability will be examined in the context of heat transfer and surface stability.
About the Speaker:
Horacio D. Espinosa is the James and Nancy Farley Professor of Manufacturing and Entrepreneurship, Professor of Mechanical Engineering, and the Director of the Theoretical and Applied Mechanics Program at the McCormick School of Engineering, Northwestern University. He received his Ph.D. in Solid Mechanics from Brown University in 1992. Espinosa has made contributions in the areas of deformation and failure of materials, design of micro- and nano-systems, in situ microscopy characterization of nanomaterials, and microfluidics for single cell manipulation and analysis. He has published over 300 technical papers in these topics. Espinosa received a number of awards including the PRAGER Medal from the Society of Engineering Science, the Society for Experimental Mechanics MURRAY and SIA NEMAT NASSER Medals, and the ASME THURSTON award. He is a member of the National Academy of Engineering (NAE), foreign member of Academia Europaea, the European Academy of Arts and Sciences, the Russian Academy of Engineering, and Fellow of AAAS, ASME, SEM, and AAM. He was the President of the Society of Engineering Science in 2012 and is a member of the IUTAM General Assembly.
Youtube Video: https://www.youtube.com/watch?v=t-d1dl5XRgw
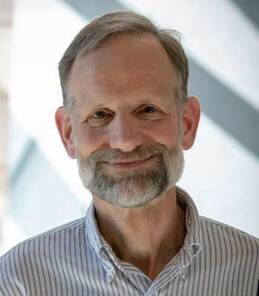
July 9 , 2021
Michael Toney, University of Colorado at Boulder
"Data Challenges in Synchrotron X-ray Scattering"
Abstract:
Over the past few decades synchrotron X-ray sources and detectors have made enormous technological gains in terms of source brightness and the widespread use of area detectors. This has resulted in a qualitative change in X-ray scattering research, as exemplified by the broad use of one-dimensional pair distribution function (PDF) or total scattering measurements. However, these advances have resulted in an explosion in amount of data that are easily obtained for any given material. There are numerous examples of these, but this talk will focus on three dimension (3D) diffuse scattering on single crystals. While this method has been used over the last several decades, recently the advances noted above have made collection fast. Consequently, this is an “emerging” method of characterizing local or short range atomic order in materials, which is increasing realized as an essential structural aspect that can determine materials function. 3D diffuse scattering denotes the broad scattering of X-rays, neutrons, and electrons “in between” Bragg diffraction peaks and this diffuse scattering encodes local order. The challenge is in the interpretation of this large volume of data and this talk will outline the challenges associated with this interpretation.
About the Speaker:
Michael Toney is a Professor of Chemical and Biological Engineering at the University of Colorado Boulder. He is a pioneer in the use of X-ray scattering and spectroscopy for the determination of atomic structure in materials for sustainable energy applications, especially solar energy and energy storage. His present interests are in hybrid metal-halide perovskites solar absorbers, Li-rich cathodes, electrode-electrolyte interfaces in energy storage systems and clean water.
Youtube Video: https://www.youtube.com/watch?v=peOKNiJXHYU
Michael Toney, University of Colorado at Boulder
"Data Challenges in Synchrotron X-ray Scattering"
Abstract:
Over the past few decades synchrotron X-ray sources and detectors have made enormous technological gains in terms of source brightness and the widespread use of area detectors. This has resulted in a qualitative change in X-ray scattering research, as exemplified by the broad use of one-dimensional pair distribution function (PDF) or total scattering measurements. However, these advances have resulted in an explosion in amount of data that are easily obtained for any given material. There are numerous examples of these, but this talk will focus on three dimension (3D) diffuse scattering on single crystals. While this method has been used over the last several decades, recently the advances noted above have made collection fast. Consequently, this is an “emerging” method of characterizing local or short range atomic order in materials, which is increasing realized as an essential structural aspect that can determine materials function. 3D diffuse scattering denotes the broad scattering of X-rays, neutrons, and electrons “in between” Bragg diffraction peaks and this diffuse scattering encodes local order. The challenge is in the interpretation of this large volume of data and this talk will outline the challenges associated with this interpretation.
About the Speaker:
Michael Toney is a Professor of Chemical and Biological Engineering at the University of Colorado Boulder. He is a pioneer in the use of X-ray scattering and spectroscopy for the determination of atomic structure in materials for sustainable energy applications, especially solar energy and energy storage. His present interests are in hybrid metal-halide perovskites solar absorbers, Li-rich cathodes, electrode-electrolyte interfaces in energy storage systems and clean water.
Youtube Video: https://www.youtube.com/watch?v=peOKNiJXHYU